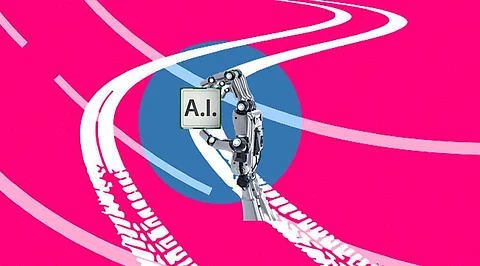
AI drift occurs when an AI system's performance and behavior change over time, often due to the evolving nature of the data it interacts with and learns from. This can result in the Artificial intelligence system making predictions or decisions that deviate from its original design and intended purpose. In essence, AI model drift is a form of algorithmic bias that can lead to unintended consequences and potentially harmful outcomes.
Unreliable Decision-Making: As AI systems drift away from their intended behavior, they may start making decisions based on outdated or incorrect assumptions. This can lead to unreliable recommendations, predictions, and actions, affecting business operations, customer interactions, and even safety-critical applications.
Ethical Concerns: AI drift can perpetuate or amplify existing biases present in training data, leading to discriminatory or unfair outcomes. For instance, a hiring AI might start favouring certain demographics or perpetuating gender or racial biases. This not only undermines diversity and fairness but can also result in legal and reputational challenges.
Safety and Security: In domains like autonomous vehicles, healthcare, and critical infrastructure, AI drift can pose significant safety risks. For instance, an AI-driven medical diagnosis system drifting in its performance might misdiagnose patients, leading to serious health consequences. Similarly, a self-driving car's behaviour changing over time could jeopardize passenger and pedestrian safety.
Regulatory Compliance: AI drift can complicate regulatory compliance efforts, as it becomes challenging to ensure that AI systems consistently adhere to industry standards and legal requirements. This can lead to increased scrutiny from regulatory bodies and potential legal liabilities.
Resource Wastage: Businesses investing substantial resources in developing and deploying AI systems could find their efforts undermined if those systems drift away from their intended functionality. This can lead to a waste of time, money, and efforts invested in AI initiatives.
Mitigating AI Drift: To address the risks associated with AI drift, proactive measures are essential:
Continuous Monitoring: Regularly monitor AI system performance and behaviour to detect any deviations from expected outcomes.
Data Quality: Ensure high-quality, diverse, and representative training data to reduce biases and decrease the likelihood of drift.
Adaptive Learning: Implement techniques that allow AI systems to adapt and learn from new data while maintaining control over changes in behaviour.
Human Oversight: Maintain human involvement and oversight in critical decision-making processes to prevent unchecked AI drift.
Feedback Loops: Establish mechanisms for receiving feedback from users and stakeholders to identify and address AI drift promptly.